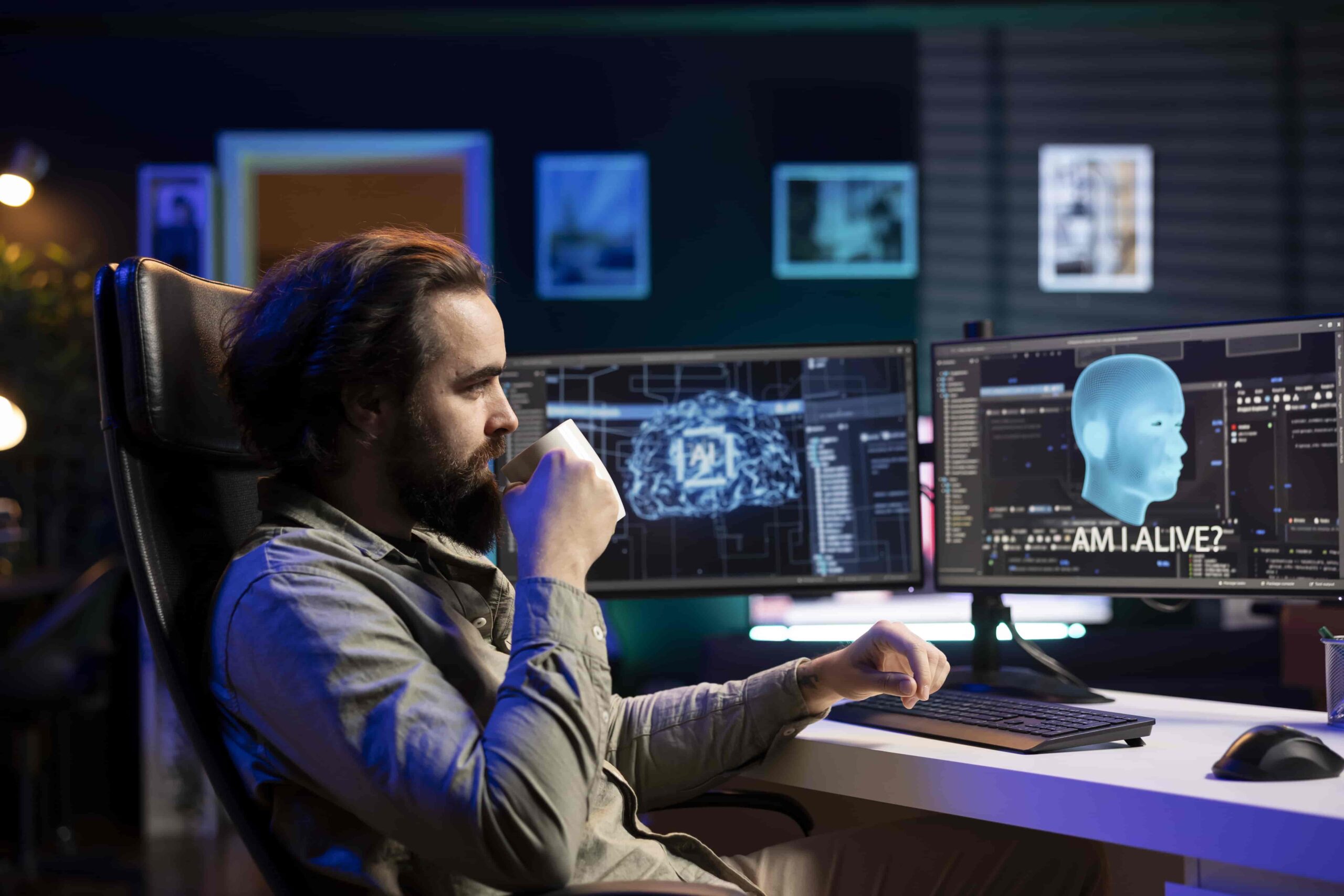
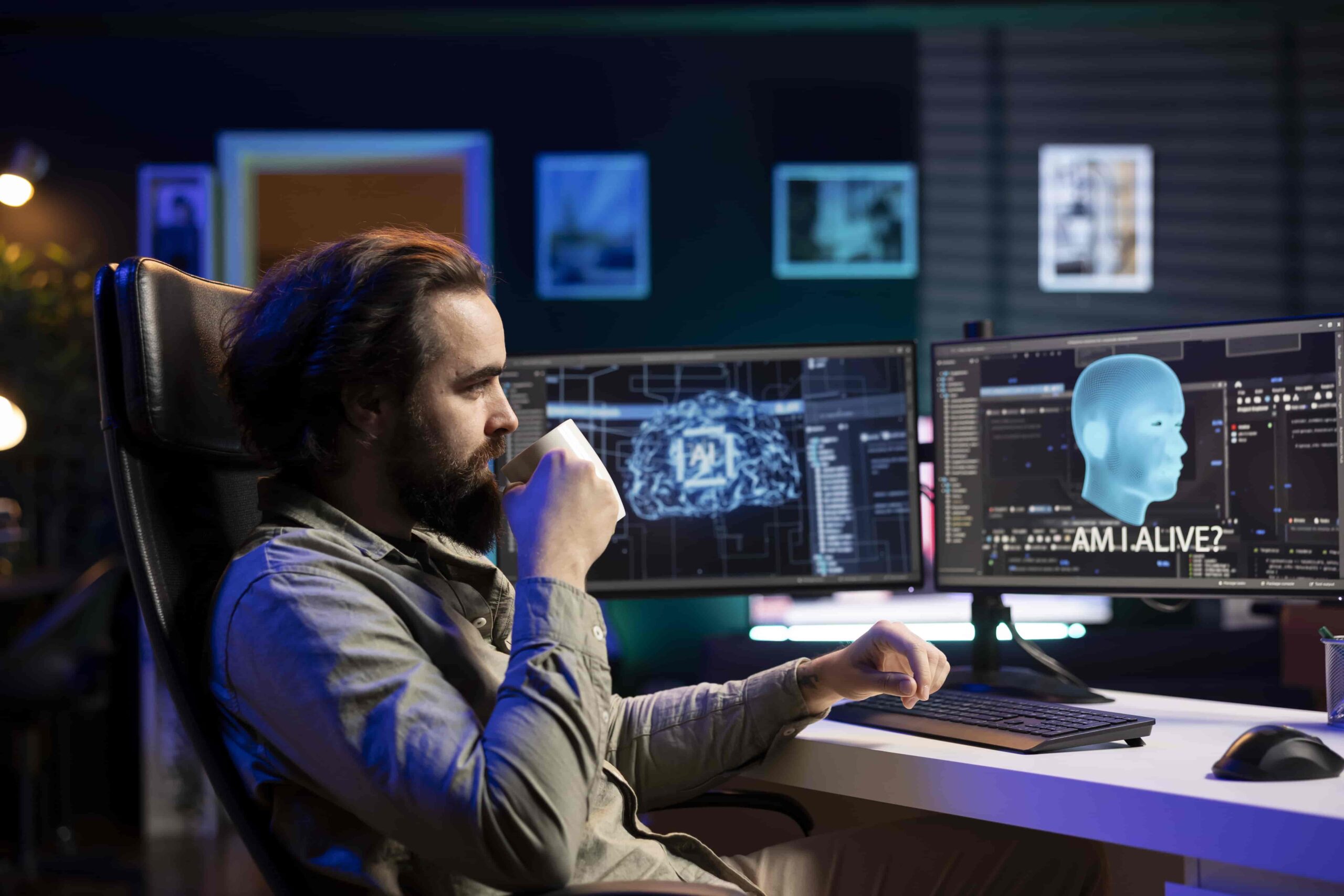
uses both transaction data and metadata to provide suggestions. It combines both content-based and collaborative models. An example is Netflix, which recommends movies based on user interest and movie features.
Companies utilizing recommendation engine software get valuable audience and user insights, important in improving operation and providing better products and services according to their needs and demands, inspiring innovation.
Creating connections is the essence of social media sites and with a recommendation engine, these sites provide consistent user engagement and a “people you might know” section to help users grow their network.
Professional-use-oriented platforms such as LinkedIn use a recommendation engine that is highly important for HR professionals looking for suitable candidates for available positions. The recommender system provides suggestions based on relevant attributes.
Get in touch with AI Consulting Group via email, on the phone, or in person.
Send us an email with the details of your enquiry including any attachments and we’ll contact you within 24 hours.
Call us if you have an immediate requirement and you’d like to chat to someone about your project needs or strategy.
We would be delighted to meet for a coffee, beer or a meal and discuss your requirements with you and your team.