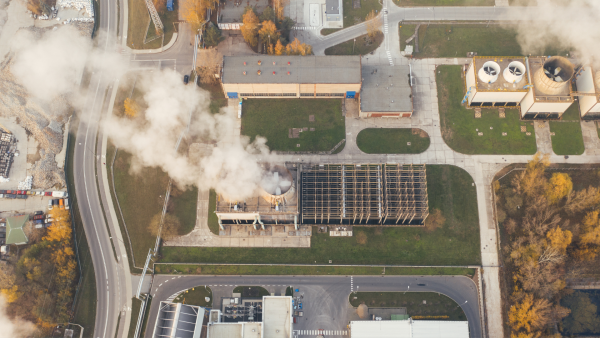
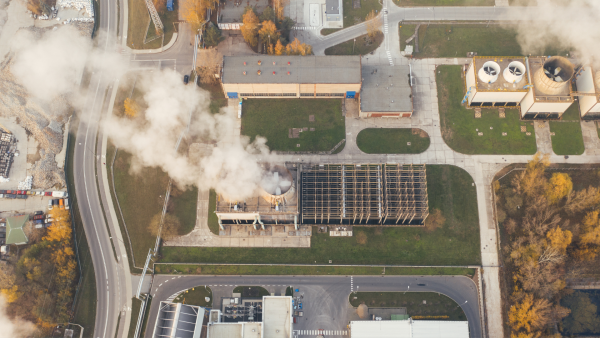
Big data is not new to the oil and gas industry. Since 1980s digital technologies has been integrated to understand reservoir’s resource and production potential. However, there has been a large gap after then and how the industry is not making the most of what technology, data, and AI in oil and gas industry.
There has been selective adoption of technology and unsystematic implementation of digitalization. To date, with more pressure on finding limited resources, there is much more potential and reason to integrate AI and ML into the operation to boost operational efficiencies, improve health and safety, and increase business value.
at large has invested proportionally less on digital and AI/ML-related technologies in the last decade compared with other sectors, such as banking, automotive, health care, retail and consumer products, and software. This is partly because AI in oil and gas industry is seen as risky, unproven technology and it requires highly skilled programmers and data scientists. But it’s also because it requires a sustained, long-term investment of dollars that many companies simply couldn’t afford during the downturn.
In addition, digital fluency and the use of AI/ML technologies have not been seen as core competencies in a sector that’s long been ruled by human operators — namely, mechanical, chemical and electrical engineers. Early adopters are typically sectors that are comfortable with digital fluency and those that have access to standardized data sets.
According to a paper by with World Economic Forum various macroeconomic, industry and technology trend is reshaping the industry now and it’s looking into a new wave of digital and business technologies. This is propelled by:
Increased consumer expectations on personalisation, engagement, speed, and sustainability are driving their energy choices. Companies are now influenced to be more transparent in their operation including hydrocarbon sources and emissions and to be more connected to multiple technology and digital platforms.
There are four themes central to the digital transformation of the oil and gas industry in the next 10 years:
The digitalization of energy systems promotes new energy sources and carriers, and supports innovative models for optimizing and marketing energy. To remain relevant to customers, the Oil and Gas industry must understand the full impact of these changes on the broader energy system.
Digital transformation in the Oil and Gas industry could unlock approximately $1.6 trillion of value for the industry, its customers, and wider society.
It could create benefits worth about $640 billion for wider society. This includes approximately $170 billion of savings for customers, roughly $10 billion of productivity improvements, $30 billion from reducing water usage and $430 billion from lowering emissions.
Environmental benefits include reducing CO2 emissions by approximately 1,300 million tonnes, saving about 800 million gallons of water, and avoiding oil spills equivalent to about 230,000 barrels of oil.
To unlock the values Data and AI in the oil and gas industry, digital transformation must be initiated from the top and embraced throughout the organisation. The World Economic Forum has six recommendations to achieve this.
Adoption of AI and ML needs to be anchored on business strategy and according to an EY study, in the context of oil and gas, the critical drivers will be:
To drive adoption, strategy must harness humans and machines to work together collectively to support scaling and creative new capabilities.
– Are there opportunities for humans and machines to work together in a new and different way?
– Can people trust technology tools to help make better decisions and add value?
– What new business capabilities is the organisation missing that it wishes to have?
AI Consulting Group can help oil and gas enterprises who wish to adopt AI in oil and gas industry. Speak to our management consultant to explore the best use cases to help your organization solve your most pressing challenges and achieve quick wins.
Get in touch with AI Consulting Group via email, on the phone, or in person.
Send us an email with the details of your enquiry including any attachments and we’ll contact you within 24 hours.
Call us if you have an immediate requirement and you’d like to chat to someone about your project needs or strategy.
We would be delighted to meet for a coffee, beer or a meal and discuss your requirements with you and your team.